- Categories: Artificial intelligence
- September 25, 2023
alexa Topical-Chat: A dataset containing human-human knowledge-grounded open-domain conversations
The responsible use of auto-replies emerges as a crucial aspect in the realm of modern communication. By setting clear expectations, addressing potential issues, and following up promptly, businesses can streamline communication channels without compromising on professionalism. Striking the right balance ensures that auto-replies enhance efficiency while maintaining the integrity of professional relationships. The landscape of chatbots is evolving at an unprecedented pace, and the open-source platforms highlighted in this comprehensive guide stand as powerhouses driving this evolution.
Wit.ai stands tall as a multifaceted NLP master, excelling in understanding nuances, breaking language barriers, and seamlessly integrating with diverse applications. As businesses seek to create chatbots that not only understand but genuinely engage with users, Wit.ai emerges as a pivotal choice in the spectrum of open-source chatbot platforms for 2024. In the dynamic landscape of open-source chatbot platforms in 2024, Verloop emerges as an exemplary force, reshaping the contours of conversational AI. The capacity for AI tools to understand sentiment and create personalized answers is where most automated chatbots today fail. Its recent progression holds the potential to deliver human-readable and context-aware responses that surpass traditional chatbots, says Tobey.
As users switch between languages, Wit.ai seamlessly adapts, providing a truly multilingual conversational experience. We introduce the Synthetic-Persona-Chat dataset, a persona-based conversational dataset, consisting of two parts. The second part consists of 5,648 new, synthetic personas, and 11,001 conversations between them. Synthetic-Persona-Chat is created using the Generator-Critic framework introduced in Faithful Persona-based Conversational Dataset Generation with Large Language Models.
They want to be doing meaningful work that really engages them, that helps them feel like they’re making an impact. And in this way we are seeing the contact center and customer experience in general evolve to be able to meet those changing needs of both the [employee experience] EX and the CX of everything within a contact center and customer experience. Shaping Answers with Rules through Conversations (ShARC) is a QA dataset which requires logical reasoning, elements of entailment/NLI and natural language generation. The dataset consists of 32k task instances based on real-world rules and crowd-generated questions and scenarios. This dataset contains over 25,000 dialogues that involve emotional situations. This is the best dataset if you want your chatbot to understand the emotion of a human speaking with it and respond based on that.
We are working on improving the redaction quality and will release improved versions in the future. If you want to access the raw conversation data, please fill out the form with details about your intended use cases. This Colab notebook provides some visualizations and shows how to compute Elo ratings with the dataset.
conversations
In this article, I will share top dataset to train and make your customize chatbot for a specific domain. We have drawn up the final list of the best conversational data sets to form a chatbot, broken down into question-answer data, customer support data, dialog data, and multilingual data. Furthermore, the API prowess of Wit.ai allows the business to integrate the chatbot with existing applications such as customer relationship management (CRM) systems, e-commerce platforms, or internal communication tools. This integration streamlines processes, enhances data flow, and creates a unified user experience across different touchpoints. Consider a scenario where a business wants to deploy a chatbot capable of understanding user queries, regardless of the language they speak. Wit.ai’s advanced NLP engine becomes the cornerstone of this capability, ensuring that the bot not only interprets the words used but also captures the nuances and context behind them.
The Dataflow scripts write conversational datasets to Google cloud storage, so you will need to create a bucket to save the dataset to. The training set is stored as one collection of examples, and
the test set as another. Examples are shuffled randomly (and not necessarily reproducibly) among the files. The train/test split is always deterministic, so that whenever the dataset is generated, the same train/test split is created. This repo contains scripts for creating datasets in a standard format –
any dataset in this format is referred to elsewhere as simply a
conversational dataset.
With powerful APIs and SDKs at their disposal, developers can take full control of the chatbot’s functionality, tailoring it to meet specific business requirements. This advanced playground caters to the needs of organisations with complex use cases, ensuring that Botpress remains a viable solution for a diverse range of industries and applications. And that while in many ways we’re talking a lot about large language models and artificial intelligence at large. Because even if we say all solutions and technologies are created equal, which is a very generous statement to start with, that doesn’t mean they’re all equally applicable to every single business in every single use case. So they really have to understand what they’re looking for as a goal first before they can make sure whatever they purchase or build or partner with is a success. I think that’s where we’re seeing those gains in conversational AI being able to be even more flexible and adaptable to create that new content that is endlessly adaptable to the situation at hand.
Models trained or fine-tuned on
This dataset contains over 8,000 conversations that consist of a series of questions and answers. You can use this dataset to train chatbots that can answer conversational questions based on a given text. Break is a set of data for understanding issues, aimed at training models to reason about complex issues. It consists of 83,978 natural language questions, annotated with a new meaning representation, the Question Decomposition Meaning Representation (QDMR). An effective chatbot requires a massive amount of training data in order to quickly resolve user requests without human intervention. However, the main obstacle to the development of a chatbot is obtaining realistic and task-oriented dialog data to train these machine learning-based systems.
It provides a challenging test bed for a number of tasks, including language comprehension, slot filling, dialog status monitoring, and response generation. QASC is a question-and-answer data set that focuses on sentence composition. It consists of 9,980 8-channel multiple-choice questions on elementary school science (8,134 train, 926 dev, 920 test), and is accompanied by a corpus of 17M sentences.
Discover how to automate your data labeling to increase the productivity of your labeling teams! Dive into model-in-the-loop, active learning, and implement automation strategies in your own projects. Investments into downsized infrastructure can help enterprises reap the benefits of AI while mitigating energy consumption, says corporate VP and GM of data center platform engineering and architecture at Intel, Zane Ball. Effective feature representations play a critical role in enhancing the performance of text generation models that rely on deep neural networks.
It is a unique dataset to train chatbots that can give you a flavor of technical support or troubleshooting. This dataset contains human-computer data from three live customer service representatives who were working in the domain of travel and telecommunications. It also contains information on airline, train, and telecom forums collected from TripAdvisor.com. This dataset contains manually curated QA datasets from Yahoo’s Yahoo Answers platform. It covers various topics, such as health, education, travel, entertainment, etc.
- The train/test split is always deterministic, so that whenever the dataset is generated, the same train/test split is created.
- If you are looking for more datasets beyond for chatbots, check out our blog on the best training datasets for machine learning.
- The 1-of-100 metric is computed using random batches of 100 examples so that the responses from other examples in the batch are used as random negative candidates.
- You can also use it to train chatbots that can answer real-world questions based on a given web document.
- Verloop’s commitment to seamless integration sets it apart in the competitive landscape of open-source chatbot platforms.
You can find more datasets on websites such as Kaggle, Data.world, or Awesome Public Datasets. You can also create your own datasets by collecting data from your own sources or using data annotation tools and then convert conversation data in to the chatbot dataset. This chatbot dataset contains over 10,000 dialogues that are based on personas. Each persona consists of four sentences that describe some aspects of a fictional character.
You can also use this dataset to train chatbots to answer informational questions based on a given text. This dataset contains over 100,000 question-answer pairs based on Wikipedia articles. You can use this dataset to train chatbots that can answer factual questions based on a given text. This dataset contains Wikipedia articles along with manually generated factoid questions along with manually generated answers to those questions. An effective chatbot requires a massive amount of training data in order to quickly solve user inquiries without human intervention. However, the primary bottleneck in chatbot development is obtaining realistic, task-oriented dialog data to train these machine learning-based systems.
Based on CNN articles from the DeepMind Q&A database, we have prepared a Reading Comprehension dataset of 120,000 pairs of questions and answers. Natural Questions (NQ), a new large-scale corpus for training and evaluating open-ended question answering systems, and the first to replicate the end-to-end process in which people find answers to questions. NQ is a large corpus, consisting of 300,000 questions of natural origin, as well as human-annotated answers from Wikipedia pages, for use in training in quality assurance systems. In addition, we have included 16,000 examples where the answers (to the same questions) are provided by 5 different annotators, useful for evaluating the performance of the QA systems learned. There are many more other datasets for chatbot training that are not covered in this article.
In the ever-evolving landscape of customer experiences, AI has become a beacon guiding businesses toward seamless interactions. While AI has been transforming businesses long before the latest wave of viral chatbots, the emergence of generative AI and large language models represents a paradigm shift in how enterprises engage with customers and manage internal workflows. HOTPOTQA is a dataset which contains 113k Wikipedia-based question-answer pairs with four key features.
Here we’ve taken the most difficult turns in the dataset and are using them to evaluate next utterance generation. This evaluation dataset contains a random subset of 200 prompts from the English OpenSubtitles 2009 dataset (Tiedemann 2009). You can download Multi-Domain Wizard-of-Oz dataset from both Huggingface and Github. This MultiWOZ dataset is available in both Huggingface and Github, You can download it freely from there. Each conversation includes a “redacted” field to indicate if it has been redacted. This process may impact data quality and occasionally lead to incorrect redactions.
- This dataset contains human-computer data from three live customer service representatives who were working in the domain of travel and telecommunications.
- In order to create a more effective chatbot, one must first compile realistic, task-oriented dialog data to effectively train the chatbot.
- Chatbot training datasets from multilingual dataset to dialogues and customer support chatbots.
- You can also use this dataset to train chatbots that can interact with customers on social media platforms.
These questions are of different types and need to find small bits of information in texts to answer them. You can try this dataset to train chatbots that can answer questions based on web documents. The objective of the NewsQA dataset is to help the research community build algorithms capable of answering questions that require human-scale understanding and reasoning skills.
As we delve into the intricate details of Botpress, it becomes evident that this open-source platform is set to redefine the chatbot experience in 2024. Rasa’s designation as an AI powerhouse is well-deserved, considering its robust NLP foundations, unparalleled customisation options, and the supportive community it has cultivated. As the demand for sophisticated chatbot solutions continues to rise, Rasa positions itself as a top contender for businesses seeking not just a chatbot but a tailored and powerful conversational AI solution. As businesses navigate the complex landscape of AI-driven communication in 2024, Verloop stands as a beacon of innovation and efficiency. Verloop’s commitment to seamless integration sets it apart in the competitive landscape of open-source chatbot platforms.
EXCITEMENT dataset… Available in English and Italian, these kits contain negative customer testimonials in which customers indicate reasons for dissatisfaction with the company. OpenBookQA, inspired by open-book exams to assess human understanding of a subject. The open book that accompanies our questions is a set of 1329 elementary level scientific facts. Approximately 6,000 questions focus on understanding these facts and applying them to new situations.
With the help of the best machine learning datasets for chatbot training, your chatbot will emerge as a delightful conversationalist, captivating users with its intelligence and wit. Embrace the power of data precision and let your chatbot embark on a journey to greatness, enriching user interactions and driving success in the AI landscape. HotpotQA is a set of question response data that includes natural multi-skip questions, with a strong emphasis on supporting facts to allow for more explicit question answering systems. NewsQA is a challenging machine comprehension dataset of over 100,000 human-generated question-answer pairs.
Some of the technologies and solutions we have can go in and find areas that are best for automation. Again, when I say best, I’m very vague there because for different companies that will mean different things. It really depends on how things are set up, what the data says and what they are doing in the real world in real time right now, what our solutions will end up finding and recommending.
Configurations were defined to impose varying degrees of
knowledge symmetry or asymmetry between partner Turkers, leading to
the collection of a wide variety of conversations. Goal-oriented dialogues in Maluuba… A dataset of conversations in which the conversation is focused on completing a task or making a decision, such as finding flights and hotels. Contains comprehensive information covering over 250 hotels, flights and destinations. This depth of understanding empowers Wit.ai powered bots to engage in more meaningful and contextually relevant conversations, enhancing the overall user experience.
The ability to deploy across diverse channels positions Botpress as an ideal solution for businesses looking to maximise their reach and engagement. The community support extends to extensive documentation, tutorials, and forums, making Rasa an accessible choice for both seasoned developers and those new to the chatbot ecosystem. But actually this is just really new technology that is opening up an entirely new world of possibility for us about how to interact with data. And so again, I say this isn’t eliminating any data scientists or engineers or analysts out there.
To quickly resolve user issues without human intervention, an effective chatbot requires a huge amount of training data. However, the main bottleneck in chatbot development is getting realistic, task-oriented conversational data to train these systems using machine learning techniques. We have compiled a list of the best conversation datasets from chatbots, broken down into Q&A, customer service data. Integrating machine learning datasets into chatbot training offers numerous advantages. These datasets provide real-world, diverse, and task-oriented examples, enabling chatbots to handle a wide range of user queries effectively. With access to massive training data, chatbots can quickly resolve user requests without human intervention, saving time and resources.
The DBDC dataset consists of a series of text-based conversations between a human and a chatbot where the human was aware they were chatting with a computer (Higashinaka et al. 2016). This dataset contains approximately 249,000 words from spoken conversations in American English. The conversations cover a wide range of topics and situations, such as family, sports, politics, education, entertainment, etc. You can use it to train chatbots that can converse in informal and casual language. This dataset contains almost one million conversations between two people collected from the Ubuntu chat logs. The conversations are about technical issues related to the Ubuntu operating system.
The user prompts are licensed under CC-BY-4.0, while the model outputs are licensed under CC-BY-NC-4.0.
The number of unique bigrams in the model’s responses divided by the total number of generated tokens. The number of unique unigrams in the model’s responses divided by the total number of generated tokens. This dataset is for the Next Utterance Recovery task, which is a shared task in the 2020 WOCHAT+DBDC. This dataset is derived from the Third Dialogue Breakdown Detection Challenge.
And again, all of this information if you have this connected system on a unified platform can then be fed into a supervisor. Recent Large Language Models (LLMs) have shown remarkable capabilities in mimicking fictional characters or real humans in conversational settings. Creating and deploying customized applications is crucial for operational success and enriching user experiences in the rapidly evolving modern business world. Our results show that SafeDecoding significantly reduces the attack success rate and harmfulness of jailbreak attacks without compromising the helpfulness of responses to benign user queries.
Datasets:
Conversational Question Answering (CoQA), pronounced as Coca is a large-scale dataset for building conversational question answering systems. You can foun additiona information about ai customer service and artificial intelligence and NLP. The goal of the CoQA challenge is to measure the ability of machines to understand a text passage and answer a series of interconnected questions that appear in a conversation. The dataset contains 127,000+ questions with answers collected from 8000+ conversations. You can use this dataset to train chatbots that can answer questions based on Wikipedia articles. We’ve put together the ultimate list of the best conversational datasets to train a chatbot, broken down into question-answer data, customer support data, dialogue data and multilingual data.
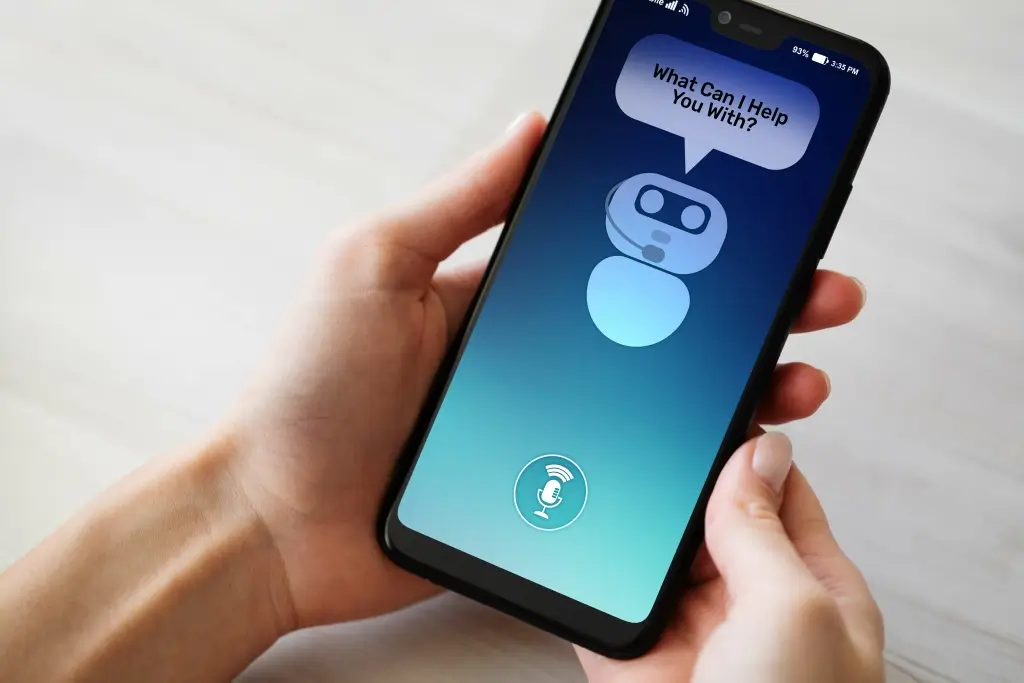
In the OPUS project they try to convert and align free online data, to add linguistic annotation, and to provide the community with a publicly available parallel corpus. SGD (Schema-Guided Dialogue) dataset, containing over 16k of multi-domain conversations covering 16 domains. Our dataset exceeds the size of existing task-oriented dialog corpora, while highlighting the challenges of creating large-scale virtual wizards.
In order to create a more effective chatbot, one must first compile realistic, task-oriented dialog data to effectively train the chatbot. Without this data, the chatbot will fail to quickly solve user inquiries or answer user questions without the need for human intervention. Lionbridge AI provides custom data for chatbot training using machine learning in 300 languages to make your conversations more interactive and support customers around the world.
DROP is a 96-question repository, created by the opposing party, in which a system must resolve references in a question, perhaps to multiple input positions, and perform discrete operations on them (such as adding, counting or sorting). These operations require a much more complete understanding of paragraph content than was required for previous data sets. Botpress distinguishes itself in the chatbot landscape with its unique blend of deployment flexibility and an advanced playground for developers. Whether you’re a business aiming for a broader audience reach, a non-technical user looking to create a basic bot, or a developer seeking advanced customisation, Botpress has the tools and features to meet your requirements. And then again, after seeing all of that information, I can continue the conversation that same way to drill down into that information and then maybe even take action to automate. And again, this goes back to that idea of having things integrated across the tech stack to be involved in all of the data and all of the different areas of customer interactions across that entire journey to make this possible.
You can also use this dataset to train a chatbot for a specific domain you are working on. TyDi QA is a set of question response data covering 11 typologically diverse languages with 204K question-answer pairs. It contains linguistic phenomena that would not be found in English-only corpora. As we venture further into 2024, Botpress stands as a testament to the evolution of open-source chatbot platforms, embodying adaptability and inclusivity. The platform’s commitment to providing a comprehensive solution for chatbot development positions it as a key player in shaping the future of conversational AI. Rasa’s strength lies in its foundation, built on state-of-the-art NLP libraries.
Top 10 Chatbot Datasets Assisting in ML and NLP Projects – Analytics Insight
Top 10 Chatbot Datasets Assisting in ML and NLP Projects.
Posted: Fri, 04 Dec 2020 08:00:00 GMT [source]
It is one of the best datasets to train chatbot that can converse with humans based on a given persona. This dataset contains automatically generated IRC chat logs from the Semantic Web Interest Group (SWIG). The chats are about topics related to the Semantic Web, such as RDF, OWL, SPARQL, and Linked Data. You can also use this dataset to train chatbots that can converse in technical and domain-specific language. Last few weeks I have been exploring question-answering models and making chatbots.
Beyond its technical prowess, Rasa takes pride in fostering a vast and active community. The strength of any open-source platform lies not just in its code but in the collective knowledge and support of its community. Rasa excels in this regard, offering a collaborative environment where developers and businesses can share insights, seek advice, and contribute to the platform’s continuous improvement. In an era where users engage across various platforms, Verloop stands out for its multichannel mastery. Businesses can reach their audience wherever they are, thanks to Verloop’s seamless integration with different communication channels. Chatbot or conversational AI is a language model designed and implemented to have conversations with humans.
You can download this Facebook research Empathetic Dialogue corpus from this GitHub link. This is the place where you can find Semantic Web Interest Group IRC Chat log dataset. Log in
or
Sign Up
to review the conditions and access this dataset content.
As businesses navigate the evolving landscape of AI-driven interactions, Rasa stands as a reliable partner, combining technical excellence with a thriving community spirit. One of Rasa’s standout features is its modular architecture, positioning it as the customisation king in the chatbot landscape. The modular structure allows developers and businesses to fine-tune every aspect of their bots.
In the fast-paced world of modern communication, auto-replies have become a valuable tool for managing incoming messages effectively. However, their implementation requires a delicate balance between automation and responsible engagement. To ensure a positive and professional interaction, it’s crucial to set clear expectations, address potential issues, and follow up promptly once available. Botpress stands out as a deployment powerhouse, showcasing remarkable flexibility by seamlessly integrating with various channels such as Facebook Messenger, Telegram, and even custom websites. This versatility ensures that your chatbot can meet your audience wherever they are, providing a unified and seamless experience across different platforms.
This adaptability ensures that businesses can tailor their chatbots to meet specific industry needs, creating a truly bespoke conversational experience for users. This dataset is created by the researchers at IBM and the University of California and can be viewed as the first large-scale dataset for QA over social media data. conversational dataset for chatbot The dataset now includes 10,898 articles, 17,794 tweets, and 13,757 crowdsourced question-answer pairs. OPUS dataset contains a large collection of parallel corpora from various sources and domains. You can use this dataset to train chatbots that can translate between different languages or generate multilingual content.
Leave a Reply